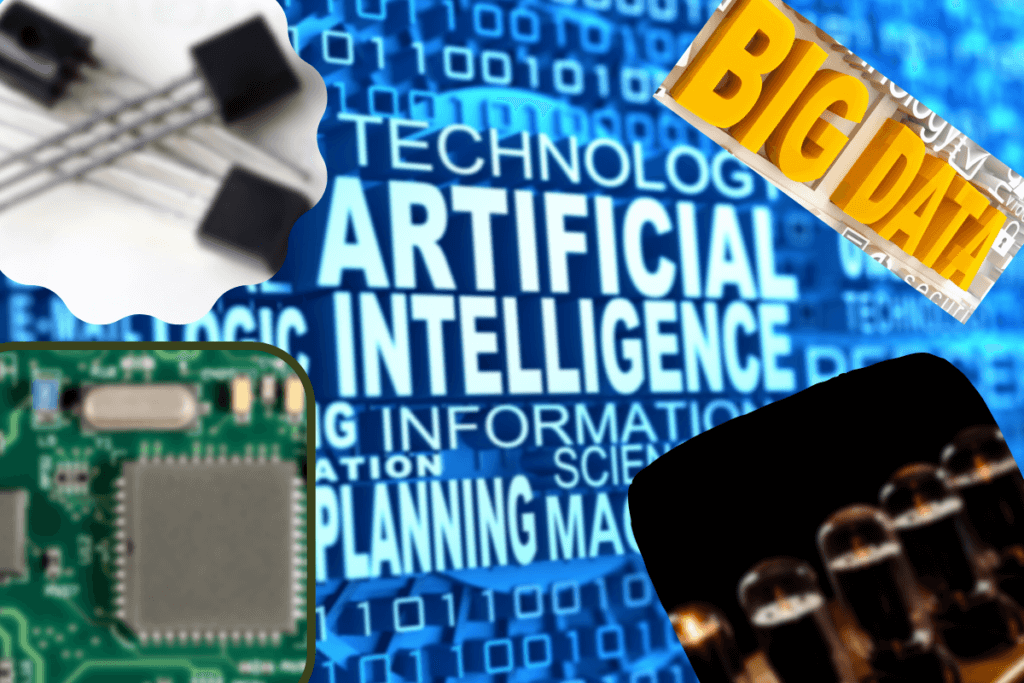
Most outrageous ideas in science are often rooted in science fiction, and artificial intelligence is no different.
Samuel Butler‘s novel Over the Range or Erewhon, first published in 1872, talked about highly developed robots with human like intelligence. He wrote that machines might develop conscience through natural selection. To be honest, natural selection of machines sounds contradictory, and probably is, but that leap of idea was very much there.
Did you notice that Erewhon is the anagram of nowhere?
But I believe Mary Shelley‘s 1818 novel Frankenstein was the first time a nonhuman with human like intelligence was written about.
In this post we will discuss Artificial Intelligence’s fascinating history and the risks and ethical issues associated with artificial intelligence.
In the early 1940s and 50s when computing machines, however huge and expensive, began to be produced, computer scientist as well as human psychologists began hoping that artificial intelligence could not be far behind.
Alan Turing, a British computer scientist introduced a game he called the imitation game in his paper “Computer Intelligence and Machinery” in 1950. In the game, one interrogator was talking to two people in two different rooms. One of the two people happened to be an AI machine posing as human. If the interrogator could not identify the machine through conversations, that machine could be called a thinking machine.
This game came to be called the Turing test and a machine that passes this test can be said to have artificial intelligence. Till date none of the machines have been able to pass this test honestly. Those who have claimed to pass were either highly specialized machines or used trickery, like chatbot Eugene Goostman in 2014, to convince the interrogators that it was a human.
The term Artificial Intelligence was coined in 1956 by John McCarthy during the Dartmouth workshop. McCarthy had organized this workshop in collaboration with other scientists hoping they would be able to lay down foundational rules for artificial intelligence but nothing much came out of it. However, governments were convinced of the power of artificial intelligence.
The Golden Years (1956 – 1974)
Researchers and artificial intelligence labs started getting funding, which enabled them to create algorithms and systems that we continue to use for training thinking machines even today.
The types of problems that these intelligent machines could solve included:
- Mathematical problems
- Word problems
- Robotics
- Machine vision
In fact, the first android or human-like robot was also designed during this period. It was a project initiated by the Waseda University, Japan, in 1967. The first humanoid robot WABOT-1 had these capabilities:
- Moving limbs that enabled it to grip and carry objects
- Eyes and ears to measure distances and direction to external objects
- Mouth to talk to others in Japanese
WABOT-1 was considered a versatile robot that could do multiple things. It was followed by WABOT-2, a specialized musician robot.
AI winter (1974-80)
Soon people got disillusioned with the promise of AI and funds started dwindling. The period between 1974 and 80 is called the AI winter on lines of nuclear winter. There were two factors responsible for the onset of AI winter:
- Researchers had tried to equate the functioning of brain to logical-mathematical algorithms – if X happens then Y else Z. But human brain turned out to be much more than logical and mathematical thinking. As you already know, humans have eight types of intelligence. So the existing algorithms were unable to replicate human intelligence, which led to disillusionment among the investors.
- The existing capacity of machines could not support the amount and speed of calculation required for the logical-mathematical algorithms developed. The computer scientists were stumped as they had to wait for hardware capacity building to check out their theory.
In hindsight, we can tell that even if we had the required computational capabilities, AI development would have bombed because most common applications like computer vision and natural language processing need humungous amount of input data, which was not available.
The Resurgence (1980-87)
Despite all odds, artificial intelligence found a resurgence in form of expert systems. Expert systems are designed to draw upon knowledge of experts in a field to provide advice or solutions to problems. The knowledge, drawn from subject matter experts, is saved in the system and when a new situation arises, the system uses artificial intelligence techniques to provide solutions. Expert systems work excellently well in highly narrowed down situations, like identifying malignant tumors, predicting onset of Parkinson’s, analyzing data to provide business insights, etc.
This resurgence was made possible due to two developments:
- Deep learning techniques that enabled computers to learn through their experience. for instance, when fully functional computer vision systems identified objects, they used it as input training data as well.
- Governments and big corporates found expert systems highly useful and hence they started funding AI research once again. For instance, the Japanese government started their Fifth Generation Computer Project and made an investment of almost $400 million over a period of eight years.
Second AI Winter (1987-93)
Expert systems are examples of narrow artificial intelligence; intelligence applied for very focused outcomes. Governments always found general artificial intelligence more enticing and useful, but expert systems could never be extrapolated to gain generalized artificial intelligence.
Commercial vendors could not develop expert systems into solutions for a wide variety of clients to ensure economic sustainability of the trend.
So once again, funds dried up and AI hype once again receded backstage. But the research scientists continues their work. By the late 1990s, chess computers began to beat chess players and grandmasters in Carnegie Mellon University and IBM labs.
The Golden Era – II (1993-2011)
Some scientists believe that the loss of hype surrounding AI was a boon in disguise for the overall AI development. As the computational power of machines increased exponentially, finally catching up with Moore’s Law, the algorithms could finally perform all calculations needed to execute any task.
Speed of a computer doubles every two years while its price is halved.
This was the era when Deep Blue defeated Gary Kasparov in 1997 and technology industry started using AI slowly but steadily. As computer power increased, AI researchers began widespread collaboration with scientists in other fields and began using refined mathematical tools to develop more refined AI systems. In many projects, the AI capabilities were part of the bigger project, but still AI moved on.
Current (2011-Now)
We now live in the age of big data. There is enough data available to train any AI system, and enough computational power to execute most complicated algorithms. Combined with deep learning, big data is taking us nearer to artificial general intelligence.
In 2017, Google’s AI machine AlphaGo defeated world’s number one Go player Ke Jie twice.
With the COVID-19 pandemic, adoption of AI technologies has accelerated by at least 7-10 years. AI capabilities are becoming such an integral part of all products and services we use that we do not think twice before using it.
Just remember that these are very narrow applications of artificial intelligence. There is so much to talk about the current phase of AI, but that’s what we will be talking about in subsequent posts.
The post appeared first on August 31, 2021
Trackbacks/Pingbacks